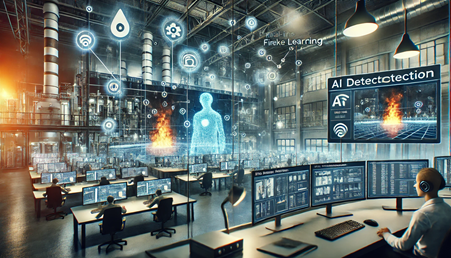
Fire detection has long been a critical component in ensuring safety in residential, commercial, and industrial settings. Traditional fire detection systems rely on smoke, heat, or flame sensors, which, while effective, often face limitations such as false alarms, delayed responses, and environmental sensitivity. With the advent of Artificial Intelligence (AI) and Machine Learning (ML), fire detection is undergoing a transformative shift. These technologies are enhancing the accuracy, speed, and efficiency of fire detection systems, reducing the risk of fire-related disasters and saving lives and property.
The Evolution of Fire Detection Systems
- Traditional Fire Detection Methods
Historically, fire detection systems have relied on:- Smoke Detectors: These devices sense smoke particles in the air. They are prone to false alarms from cooking, steam, or dust.
- Heat Detectors: Activated by an increase in temperature, heat detectors are slower to respond compared to smoke detectors, making them less suitable for early fire detection.
- Flame Detectors: These detect flames through infrared or ultraviolet light but require a clear line of sight, limiting their applicability in certain environments.
- Limitations of Traditional Systems
Traditional systems have several drawbacks, including:- False Alarms: High rates of false alarms lead to complacency, where real alarms may be ignored.
- Delayed Response: Delays in detection can result in significant fire damage before intervention.
- Environmental Sensitivity: Smoke and heat detectors can be affected by environmental conditions like humidity, temperature changes, or dust, leading to unreliable performance.
The Role of AI and Machine Learning in Fire Detection
AI and ML are revolutionizing fire detection by addressing the limitations of traditional systems and introducing new capabilities.
- Enhanced Accuracy and Reduced False Alarms
AI and ML algorithms analyze data from various sensors and cameras, learning to distinguish between actual fire incidents and false positives. By considering multiple factors such as temperature patterns, smoke density, and visual cues, these systems significantly reduce false alarms. - Faster Detection and Response
ML models can detect subtle signs of fire that may not trigger traditional detectors. For example, AI-powered video surveillance can identify smoke or flame patterns before they become visible to the human eye, enabling faster response times and reducing the potential for fire spread. - Integration with IoT Devices
AI-powered fire detection systems can integrate with the Internet of Things (IoT) devices, creating a connected network of sensors, cameras, and control systems. This integration allows for real-time monitoring and automated responses, such as activating sprinklers, shutting down electrical systems, or notifying emergency services.
Key Technologies in AI-Powered Fire Detection
- Computer Vision
Computer vision technology enables fire detection systems to analyze visual data from cameras. By applying ML algorithms, these systems can identify smoke, flames, or unusual heat patterns in real-time, even in challenging environments. - Neural Networks
Deep learning models, particularly convolutional neural networks (CNNs), are used to process and interpret complex data. These networks are trained on vast datasets of fire-related images and scenarios, allowing them to accurately detect fire events in new, unseen situations. - Data Fusion
AI systems can fuse data from multiple sources, such as thermal cameras, smoke detectors, and environmental sensors. This multi-sensor approach enhances the reliability of fire detection, as the system cross-verifies information before issuing an alert.
Applications of AI and ML in Fire Detection
- Smart Homes
AI-enabled fire detection systems are becoming an integral part of smart home ecosystems. These systems provide homeowners with real-time alerts on their smartphones, allowing for immediate action even when they are away. - Industrial and Commercial Settings
In industrial environments, AI-based systems monitor high-risk areas such as chemical plants, oil refineries, and manufacturing facilities. These systems not only detect fires but also predict potential fire hazards by analyzing historical data and identifying patterns that precede fire incidents. - Forest Fire Detection
AI and ML are playing a crucial role in combating forest fires. Satellite imagery, drone footage, and sensor data are analyzed in real-time to detect early signs of wildfires. This proactive approach helps in containing fires before they spread uncontrollably, protecting vast areas of forest and wildlife.
Challenges and Future Prospects
- Challenges
- Data Quality and Availability: Training ML models requires large datasets of fire incidents. Ensuring the availability and quality of such data can be challenging.
- Complexity and Cost: Implementing AI-based systems involves complex algorithms and infrastructure, which can be costly and require specialized expertise.
- Regulatory and Ethical Concerns: The deployment of AI in fire detection raises concerns about privacy, data security, and the potential for over-reliance on automated systems.
- Future Prospects
Despite the challenges, the future of AI in fire detection looks promising. Advancements in AI and ML will lead to even more sophisticated and reliable systems. Key areas of development include:- Predictive Analytics: Using AI to predict fire outbreaks by analyzing environmental conditions, human activities, and historical data.
- Edge Computing: Deploying AI models on edge devices for faster processing and reduced reliance on centralized systems.
- Global Collaboration: Sharing data and insights across borders to improve AI algorithms and enhance global fire detection capabilities.
Conclusion
AI and Machine Learning are transforming fire detection by making it more accurate, faster, and responsive. These technologies are not only reducing the risk of fire-related disasters but also paving the way for smarter, more connected safety systems. As AI continues to evolve, its impact on fire detection will only grow, ensuring greater safety and security for communities worldwide.
Leave A Comment